1. Introduction
Bayesian networks are powerful graphical models that represent probabilistic relationships among a set of variables. They are used in various fields, from medical diagnosis to financial risk assessment, allowing practitioners to make informed decisions under uncertainty. This article will explore the fundamental concepts of Bayesian networks, their workings, real-world applications, and challenges, along with illustrative examples.
2. Fundamental Concepts of Bayesian Networks
2.1. What is a Bayesian Network?
A Bayesian network is a directed acyclic graph (DAG) consisting of nodes and directed edges. Each node represents a variable, and the edges signify direct dependencies between these variables. The absence of an edge between two nodes indicates a conditional independence, meaning that the knowledge of one node provides no information about the other, given the knowledge of their parent nodes.
2.2. Conditional Probability Tables (CPTs)
Conditional Probability Tables (CPTs) are essential components of Bayesian networks that quantify the relationships between variables. Each node has an associated CPT that specifies the probability of each possible value of the node, conditioned on the values of its parent nodes. This allows the network to model complex dependencies in a structured manner.
3. How Bayesian Networks Work
3.1. Structure of a Bayesian Network
The structure of a Bayesian network is crucial for accurately representing relationships among variables. For example, in a network modeling weather conditions, nodes may represent factors such as “Rain,” “Traffic,” and “Accidents.” The edges illustrate how weather influences traffic patterns and how traffic can impact accident rates.
3.2. Inference in Bayesian Networks
Inference in Bayesian networks involves calculating the probabilities of certain outcomes given observed evidence. This can be done through exact methods, such as variable elimination, or approximate methods, like Monte Carlo simulations. For instance, if we know it is raining, we can use the network to infer the increased likelihood of traffic congestion and accidents.

4. Real-World Applications of Bayesian Networks
4.1. Medical Diagnosis
One of the prominent applications of Bayesian networks is in medical diagnosis. For example, consider a Bayesian network that diagnoses diseases based on symptoms and laboratory test results. Nodes could include “Flu,” “Cough,” and “Fever.” By inputting observed symptoms, the network can update the probabilities of different diseases, aiding healthcare professionals in making accurate diagnoses.
4.2. Risk Assessment in Finance
Bayesian networks are also valuable in finance for assessing risks associated with investments. For instance, a network might model factors such as “Market Conditions,” “Company Performance,” and “Economic Indicators.” By analyzing the relationships between these variables, investors can evaluate potential risks and make informed investment decisions. A real-world case study could involve evaluating the risk of investing in a startup based on market trends and financial health.
4.3. Decision Support Systems
In decision-making processes, Bayesian networks serve as decision support systems. They can integrate various factors affecting a decision, such as resource allocation in healthcare. For example, a network may model the relationships between patient outcomes, available treatments, and hospital resources, helping administrators optimize resource distribution to improve patient care.
5. Building a Bayesian Network
5.1. Steps to Construct a Bayesian Network
To build a Bayesian network, one must:
- Identify Variables: Determine relevant variables that influence each other.
- Define Relationships: Establish directed edges to represent dependencies.
- Specify Conditional Probabilities: Create CPTs for each variable based on prior knowledge or data.
5.2. Tools for Building Bayesian Networks
Several software tools can assist in modeling Bayesian networks, such as Netica, GeNIe, and BayesiaLab. These tools offer user-friendly interfaces for defining structures, entering probabilities, and performing inference, making them accessible for practitioners and researchers alike.
6. Challenges and Limitations
6.1. Data Requirements
Bayesian networks require sufficient data to accurately define relationships and probabilities. In domains with limited data, it can be challenging to establish reliable CPTs, potentially leading to inaccuracies in predictions.
6.2. Complexity in Large Networks
As the number of variables increases, the complexity of Bayesian networks can grow exponentially. Managing this complexity while maintaining interpretability is a significant challenge. Techniques such as model simplification and hierarchical modeling can help mitigate these issues.
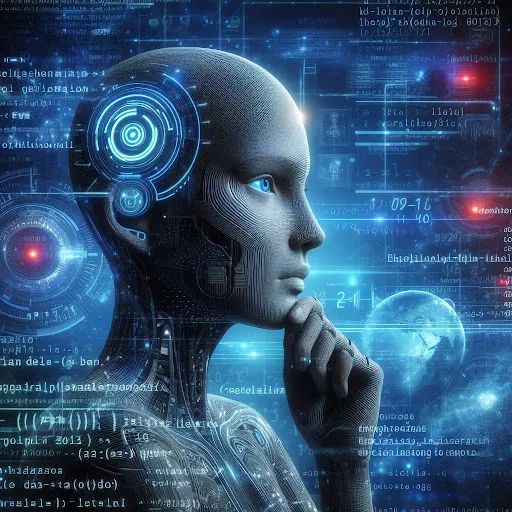
7. Future Directions of Bayesian Networks
7.1. Integration with Machine Learning
The convergence of Bayesian networks and machine learning is an exciting area for future research. Integrating Bayesian principles with deep learning can enhance model robustness and interpretability, allowing for better handling of uncertainty in predictions.
7.2. Advances in Computational Techniques
Ongoing advancements in algorithms and computational techniques continue to improve the scalability of Bayesian networks. New methods, such as variational inference, enable efficient approximations of posterior distributions, making Bayesian networks applicable to larger datasets and more complex problems.
8. Conclusion
Bayesian networks offer a robust framework for modeling uncertainty and representing probabilistic relationships among variables. Their ability to incorporate prior knowledge and update beliefs based on new evidence makes them invaluable across various fields, from healthcare to finance. Understanding Bayesian networks and their applications can empower practitioners to make informed decisions in an uncertain world.
9. References
- A comprehensive list of academic papers, textbooks, and online resources for further reading on Bayesian networks and their applications in various domains.
FAQs
1. What are Bayesian networks?
Bayesian networks are graphical models that represent probabilistic relationships among a set of variables using directed acyclic graphs (DAGs). They consist of nodes (representing variables) and edges (indicating dependencies).
2. How do Bayesian networks work?
Bayesian networks work by utilizing conditional probability tables (CPTs) that quantify the relationships between variables. They perform inference to update the probabilities of certain outcomes based on observed evidence.
3. What are Conditional Probability Tables (CPTs)?
CPTs are tables associated with each node in a Bayesian network that specify the probability of each possible state of the node, given the states of its parent nodes. They are crucial for modeling the dependencies in the network.

4. What are some real-world applications of Bayesian networks?
Bayesian networks are used in various applications, including:
- Medical Diagnosis: Diagnosing diseases based on symptoms and test results.
- Risk Assessment in Finance: Evaluating investment risks based on market conditions and company performance.
- Decision Support Systems: Assisting in decision-making processes, such as resource allocation in healthcare.
5. What are the steps to build a Bayesian network?
To build a Bayesian network, follow these steps:
- Identify the relevant variables.
- Define the relationships between the variables.
- Specify the conditional probabilities using CPTs.
6. What tools are available for constructing Bayesian networks?
Several software tools can aid in building Bayesian networks, including:
- Netica
- GeNIe
- BayesiaLab
These tools offer user-friendly interfaces for modeling and inference.
7. What are the challenges associated with Bayesian networks?
Challenges include:
- Data Requirements: The need for sufficient data to accurately define relationships and probabilities.
- Complexity in Large Networks: As the number of variables increases, managing the complexity of the network can become challenging.
8. How do Bayesian networks relate to machine learning?
Bayesian networks can enhance machine learning models by providing a framework for handling uncertainty and incorporating prior knowledge. Their integration with deep learning techniques is a promising area for future research.
9. What is the future direction of Bayesian networks?
Future directions include advancements in computational techniques that improve scalability and efficiency. There is also potential for integrating Bayesian networks with emerging technologies in machine learning.
Tips for Understanding and Using Bayesian Networks
- Start with Simple Models: If you’re new to Bayesian networks, begin with simple models to understand the basic concepts of nodes, edges, and CPTs before tackling more complex networks.
- Leverage Software Tools: Utilize software tools like Netica or GeNIe to visualize and build your Bayesian networks. These tools simplify the modeling process and allow for efficient inference.
- Study Real-World Examples: Analyze existing Bayesian networks used in real-world applications to gain insights into their structure and functioning. This can provide practical understanding and inspiration for your own projects.
- Experiment with Different Data: To grasp the impact of data on Bayesian networks, experiment with different datasets and observe how changes affect the probabilities and inferences.
- Engage with the Community: Join online forums or communities focused on Bayesian statistics and machine learning. Engaging with others can help you learn from their experiences and share your knowledge.
- Document Your Models: As you create Bayesian networks, document the assumptions and choices made, especially regarding prior distributions and relationships. This practice will help in understanding and refining the model later.
- Validate Your Models: Whenever possible, validate your Bayesian models against real-world outcomes to assess their accuracy and reliability. This feedback loop is crucial for improving model performance.
- Stay Updated: Keep abreast of the latest research and advancements in Bayesian methods. Online courses, webinars, and academic papers can provide valuable insights and techniques.