1. Introduction
In an age overflowing with information, the ability to distill vast amounts of text into concise summaries is invaluable. Text summarization, a critical aspect of Natural Language Processing (NLP), automates this task by generating a shortened version of a text while retaining its essential meaning. This article explores various text summarization techniques, focusing on their applications, advantages, and limitations.
2. Types of Text Summarization
Text summarization can be broadly classified into two categories: extractive and abstractive summarization.
2.1. Extractive Summarization
Extractive summarization involves selecting key sentences or phrases directly from the source text. The goal is to identify the most significant parts of the text and piece them together to form a coherent summary.
- Advantages:
- Simplicity: The method is straightforward and easy to implement.
- Preservation of Original Wording: Since sentences are selected directly, the original phrasing and structure are retained.
- Limitations:
- Lack of Coherence: The extracted sentences may not flow well together.
- Surface-Level Understanding: It may miss the underlying context or nuanced meanings.
2.2. Abstractive Summarization
In contrast, abstractive summarization generates new sentences that capture the essence of the original text. This approach aims to produce summaries that paraphrase the source material rather than merely extracting it.
- Advantages:
- Greater Coherence: Abstractive summaries can be crafted to flow better and present information more logically.
- Enhanced Understanding: This method can convey complex ideas more effectively by reformulating the original text.
- Limitations:
- Complexity: Developing abstractive models requires more sophisticated algorithms and training data.
- Risk of Inaccuracy: The generated text may misrepresent the original content or introduce factual errors.
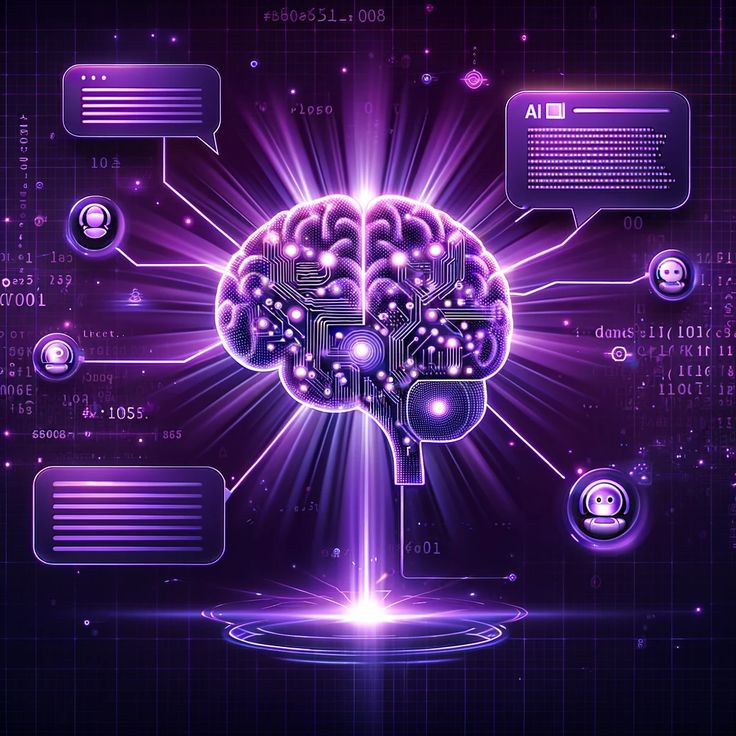
3. Techniques for Extractive Summarization
Several techniques can be employed for extractive summarization, including:
3.1. Frequency-Based Methods
Frequency-based methods assess the importance of sentences based on the frequency of significant words. One popular technique is Term Frequency-Inverse Document Frequency (TF-IDF), which weighs terms based on their frequency in a document relative to their frequency across a collection of documents. Sentences are ranked based on the cumulative TF-IDF scores of their words.
3.2. Graph-Based Methods
Graph-based methods, such as TextRank, utilize graph representations of sentences. Each sentence is treated as a node, and edges represent the similarity between sentences based on shared words. The algorithm ranks sentences using centrality measures, selecting the top-ranked sentences for inclusion in the summary.
3.3. Machine Learning Approaches
Supervised machine learning techniques can also be applied to extractive summarization. In this approach, features are extracted from sentences, and a classification model is trained to distinguish between important and unimportant sentences. Popular algorithms include Support Vector Machines (SVM) and Decision Trees.
4. Techniques for Abstractive Summarization
Abstractive summarization employs various advanced techniques, such as:
4.1. Sequence-to-Sequence Models
Sequence-to-sequence (seq2seq) models are fundamental in abstractive summarization. These models use an encoder-decoder architecture, where the encoder processes the input text and the decoder generates the summary. This architecture enables the model to learn relationships between input sequences and their corresponding outputs.
4.2. Transformer Models
Transformers, like BERT and GPT, have revolutionized NLP by providing a robust framework for generating high-quality abstractive summaries. These models utilize self-attention mechanisms, allowing them to capture contextual information effectively. By fine-tuning transformer models on summarization tasks, they can generate coherent and contextually relevant summaries.
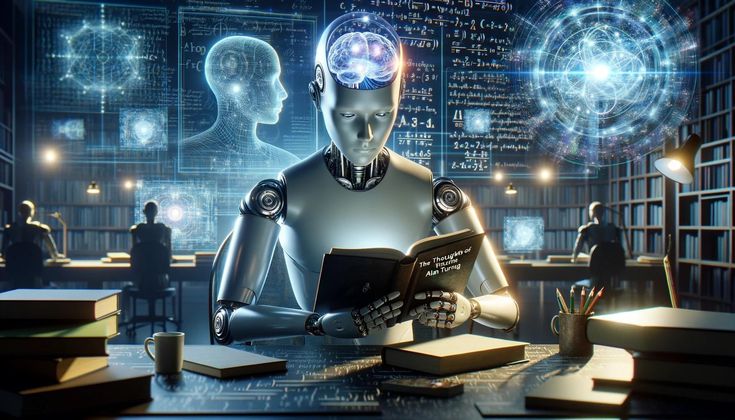
4.3. Reinforcement Learning
Reinforcement learning techniques can enhance summarization models by incorporating reward mechanisms based on the quality of the generated summaries. By evaluating outputs against reference summaries using metrics like ROUGE, the model learns to optimize its summarization strategy over time.
5. Evaluation Metrics for Summarization
Evaluating summarization quality is crucial to determine its effectiveness. Common evaluation metrics include:
- ROUGE: A set of metrics that measure the overlap between the generated summary and reference summaries. ROUGE-N (precision, recall, and F1 score for n-grams) is particularly popular.
- BLEU: Although primarily used in machine translation, BLEU measures the precision of n-grams in the generated summary compared to reference summaries.
- Human Evaluation: In addition to automated metrics, human evaluation provides qualitative assessments of summary coherence, relevance, and informativeness.
Selecting appropriate metrics depends on the summarization type and intended application.
6. Applications of Text Summarization
Text summarization has numerous applications across various domains:
- News Summarization: Automatically summarizing articles helps readers quickly grasp current events.
- Document Summarization: Legal and academic fields utilize summarization tools to distill lengthy documents into key points for easier understanding.
- Customer Support Systems: Summarization aids in generating concise responses to customer inquiries by analyzing previous interactions.
- Real-Time Social Media Summarization: In platforms like Twitter, summarization algorithms can help condense trending topics or events into digestible insights.
7. Challenges and Future Directions
Despite significant advancements, text summarization faces several challenges:
- Handling Ambiguity: The complexity of natural language often leads to ambiguities that can confuse summarization models.
- Bias and Misrepresentation: Summarization models may inadvertently reflect biases present in their training data, leading to inaccurate or biased summaries.
- Need for Better Contextual Understanding: Enhancing the ability of models to capture nuanced meanings and context is an ongoing research focus.
Future directions include developing more sophisticated models, integrating multi-modal inputs (text, images, etc.), and improving evaluation methods to assess summary quality more effectively.
8. Conclusion
Text summarization is a vital component of NLP that aids in managing information overload. By understanding the various techniques employed in extractive and abstractive summarization, researchers and practitioners can enhance their applications across different domains. As technology continues to evolve, the potential for more effective and accurate summarization methods remains promising, paving the way for improved communication and information accessibility.
9. References
- Liu, Y., & Lapata, M. (2019). “Text Summarization with Pretrained Encoders.”
- Nallapati, R., et al. (2016). “Abstractive Text Summarization using Sequence-to-Sequence RNNs and Beyond.”
- Chen, J., & Bansal, M. (2018). “Hugging Face’s Transformers: State-of-the-art Natural Language Processing.”
- Zhang, J., et al. (2020). “Pre-trained Models for Text Summarization: A Review.”
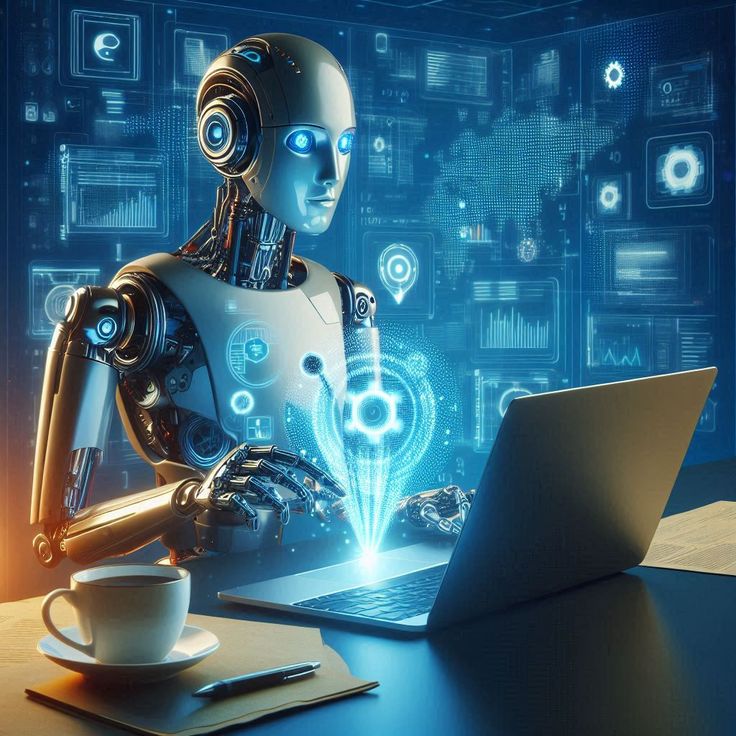
FAQs
1. What is text summarization?
Text summarization is the process of reducing a text document to its essential information, creating a concise version that retains the original meaning. It is a key area in Natural Language Processing (NLP) used to facilitate information retrieval and comprehension.
2. What are the two main types of text summarization?
The two main types are:
- Extractive Summarization: Selects and compiles key sentences or phrases from the original text.
- Abstractive Summarization: Generates new sentences that capture the essence of the original text, often rephrasing and condensing information.
3. What are some common techniques used in extractive summarization?
Common techniques include:
- Frequency-Based Methods: Using metrics like TF-IDF to identify important sentences.
- Graph-Based Methods: Utilizing algorithms like TextRank to rank sentences based on their relationships.
- Machine Learning Approaches: Applying supervised learning models to classify and extract key sentences.
4. What advanced techniques are used in abstractive summarization?
Techniques include:
- Sequence-to-Sequence Models: Using encoder-decoder architectures for generating summaries.
- Transformer Models: Employing models like BERT and GPT that leverage self-attention mechanisms for contextual understanding.
- Reinforcement Learning: Implementing reward-based strategies to improve summarization quality.
5. How is the quality of summarization evaluated?
The quality of summarization is typically evaluated using metrics such as:
- ROUGE: Measures the overlap between the generated and reference summaries.
- BLEU: Primarily used in machine translation, it assesses the precision of generated n-grams.
- Human Evaluation: Involves qualitative assessments to judge coherence, relevance, and informativeness.
6. What are some practical applications of text summarization?
Text summarization is applied in various domains, including:
- News articles summarization.
- Legal and academic document summarization.
- Customer support systems for generating concise responses.
- Real-time summarization of social media content.
7. What challenges does text summarization face?
Challenges include:
- Handling ambiguity and complexity in natural language.
- Addressing bias and misrepresentation in generated summaries.
- Improving contextual understanding to capture nuanced meanings.
8. What is the future direction for text summarization research?
Future directions may include developing more sophisticated models, integrating multi-modal inputs, enhancing evaluation methods, and addressing ethical concerns related to summarization outputs.
9. How can I get started with text summarization?
You can start by:
- Exploring existing summarization libraries like Hugging Face Transformers.
- Experimenting with different algorithms on sample datasets.
- Learning from tutorials and online courses focused on NLP and summarization techniques.
10. Are there any ethical considerations in text summarization?
Yes, ethical considerations include:
- Ensuring the generated summaries do not propagate biases present in training data.
- Maintaining accuracy and reliability in summarizing sensitive or critical information.
Tips for Understanding Text Summarization Techniques
- Familiarize with NLP Basics: Understanding fundamental concepts in NLP will help you grasp summarization techniques better.
- Experiment with Tools: Utilize existing NLP libraries like NLTK, SpaCy, or Hugging Face to practice text summarization on real datasets.
- Read Research Papers: Engage with foundational and recent research in summarization to stay updated on advancements in the field.
- Participate in Online Courses: Take advantage of MOOCs that focus on NLP, deep learning, and specifically text summarization.
- Engage in Community Discussions: Join online forums or communities to discuss challenges and insights related to text summarization.
- Work on Practical Projects: Build projects that implement summarization techniques to solidify your understanding and gain hands-on experience.
- Stay Informed on Best Practices: Follow blogs and articles on the latest trends, techniques, and ethical practices in text summarization.
- Seek Feedback: When working on summarization tasks, share your results with peers to get constructive feedback on improvement areas.
- Experiment with Different Metrics: Evaluate summarization quality using various metrics to understand their strengths and weaknesses.
- Focus on Ethical Usage: Always consider the implications of summarization outputs, especially when dealing with sensitive content or diverse audiences.