Generative models, a cornerstone of modern artificial intelligence, have opened up new realms of creativity across various fields. One of the most exciting applications is in music, where these models are reshaping how music is composed, experienced, and produced. This article explores the innovative ways generative models are being used in music, illustrating their impact on both the creation and consumption of sound.
1. Introduction
The advent of generative models has revolutionized creative industries by enabling machines to learn and mimic patterns from existing data. In music, these models analyze vast amounts of musical compositions to generate new pieces, allowing artists to push the boundaries of their creative processes. Exploring the intersection of generative models and music reveals a plethora of innovative applications that challenge traditional notions of music creation.
2. Understanding Generative Models in Music
Definition of Generative Models
Generative models refer to algorithms that learn the underlying distribution of a dataset, allowing them to generate new data instances resembling the original. In music, these models can create new compositions by understanding and replicating musical structures, styles, and elements.
Types of Generative Models Used in Music
- Variational Autoencoders (VAEs): These models encode musical data into a compressed representation, enabling the generation of new compositions that share characteristics with the training data.
- Generative Adversarial Networks (GANs): Comprising a generator and a discriminator, GANs can create realistic-sounding music by competing against each other to produce high-quality outputs.
- Recurrent Neural Networks (RNNs) and Long Short-Term Memory (LSTM) Networks: These models are particularly suited for sequential data, making them effective for music generation, as they can remember and leverage previous notes or chords.
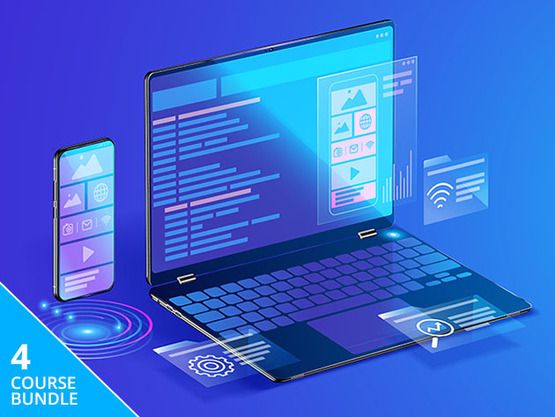
3. Automated Composition
Algorithmic Music Composition
Generative models can autonomously compose original pieces of music, using learned patterns from existing works. These models can produce a wide range of genres and styles, from classical symphonies to contemporary pop.
Examples of Tools and Platforms
Notable applications like OpenAI’s MuseNet and Google’s Magenta demonstrate the potential of generative models in music composition. MuseNet, for instance, can generate complex musical compositions in various styles, integrating different instruments and genres.
Benefits and Limitations
While generative models can produce impressive compositions, they may lack the emotional depth and nuanced understanding that human composers bring to their work. This raises questions about the authenticity of AI-generated music.
4. Music Style Transfer
Definition and Process
Generative models can also facilitate music style transfer, allowing a piece to retain its melody while adopting the stylistic elements of another genre. This involves training the model to recognize different styles and apply them to new compositions.
Applications
An example of music style transfer is transforming a classical piece into a jazz interpretation. This process enriches the music landscape by blending various genres, leading to unique creations.
Impact on Music Creation
By enabling cross-genre experimentation, generative models foster innovation in music creation, allowing artists to explore new soundscapes and reach diverse audiences.
5. Sound Design and Synthesis
Creating Unique Sounds
Generative models can be employed to design novel sounds and textures that are not easily created through traditional means. These unique sounds can become integral components of new musical compositions.
Applications in Electronic Music
Many electronic music producers leverage generative models for sound synthesis, incorporating AI-generated sounds into their tracks to create distinctive auditory experiences.
Tools for Sound Generation
Software and plugins like Serum and Pure Data utilize generative models for sound design, providing musicians with innovative tools to explore new sonic possibilities.
6. Interactive Music Systems
Generative Music Applications
Generative models can create music that evolves based on user interaction. This interactivity allows listeners to engage with music in novel ways, tailoring their experiences in real-time.
Examples of Interactive Installations
Art installations, such as generative soundscapes in galleries, invite audiences to experience music as an evolving entity rather than a static performance. These installations often respond to environmental factors or audience movements.
Impact on Audience Experience
By engaging audiences in dynamic ways, generative music systems create immersive experiences, transforming how people perceive and interact with music.
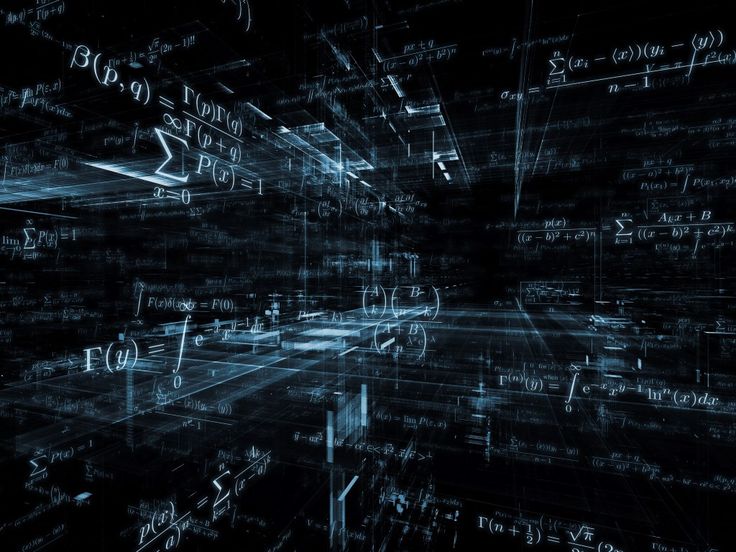
7. Music Recommendation and Personalization
Generative Models for Recommendation Systems
Generative models can enhance music recommendation algorithms by analyzing listener preferences and generating tailored playlists that introduce users to new artists and songs they may enjoy.
Personalized Music Generation
Beyond recommendations, generative models can create personalized music based on individual listeners’ tastes, generating unique tracks that resonate with their emotional states or preferences.
Implications for Music Discovery
This personalized approach revolutionizes music discovery, helping listeners explore new genres and artists in ways that align with their unique preferences.
8. Collaboration Between Humans and AI
Co-Creation Models
Generative models enable collaborative music creation between human artists and AI, fostering a partnership where both parties contribute to the creative process.
Examples of Successful Collaborations
Notable projects, such as collaborations between musicians and AI systems like AIVA (Artificial Intelligence Virtual Artist), showcase the potential of AI to enhance human creativity, blending the strengths of both.
Future of Human-AI Collaboration in Music
As generative models evolve, the relationship between musicians and AI is likely to deepen, leading to more innovative and diverse musical expressions.
9. Challenges and Ethical Considerations
Quality vs. Authenticity
The rise of AI-generated music raises questions about the quality and authenticity of compositions. Some argue that AI lacks the emotional depth and context that human composers provide.
Copyright Issues
Navigating copyright rights for AI-generated music poses challenges. Determining ownership and rights for music created collaboratively by humans and AI is an ongoing debate in the industry.
Cultural Implications
Generative music can impact cultural traditions, as AI systems might inadvertently overlook the nuances and significance of specific musical forms and styles.
10. Future Trends in Generative Music
Advancements in Technology
The rapid advancement of machine learning and AI will lead to more sophisticated generative models capable of producing high-quality music across various genres.
Potential Applications
Future applications may include more personalized music experiences, enhanced interactive installations, and deeper integration of generative models into live performances.
Role in Music Education
Generative models could play a significant role in music education, offering students innovative tools for composition and analysis, helping them learn music theory and composition in engaging ways.
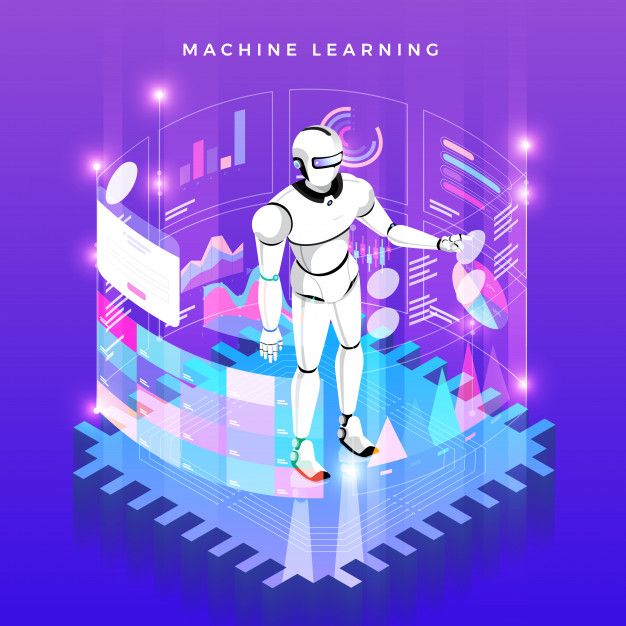
11. Conclusion
The creative uses of generative models in music are reshaping the landscape of music creation, performance, and consumption. As these technologies continue to evolve, they will likely inspire new forms of artistic expression and challenge our understanding of creativity in music.
FAQs: Creative Uses of Generative Models in Music
1. What are generative models in music?
Generative models are algorithms that learn from existing musical data to create new compositions or sounds. They can replicate styles, generate melodies, or produce entire pieces of music autonomously.
2. How do generative models compose music?
These models analyze vast datasets of music to understand patterns, structures, and styles. They then use this knowledge to create new compositions that resemble the training data, allowing for diverse musical outputs.
3. What are the main types of generative models used in music?
The primary types include:
- Variational Autoencoders (VAEs): Focus on encoding and reconstructing music.
- Generative Adversarial Networks (GANs): Use a competitive approach between a generator and a discriminator to produce realistic music.
- Recurrent Neural Networks (RNNs): Utilize sequential data processing, ideal for musical compositions.
4. What are some applications of generative models in music?
Generative models are used for:
- Automated music composition.
- Music style transfer.
- Sound design and synthesis.
- Interactive music systems that respond to user input.
- Music recommendation and personalization.
5. How do generative models enhance sound design?
Generative models allow musicians to create unique sounds and textures that may not be achievable through traditional means, contributing to the development of new and innovative music.
6. What challenges are associated with using generative models in music?
Challenges include ensuring the quality and emotional depth of AI-generated music, navigating copyright issues, and addressing cultural implications related to music authenticity and representation.
7. Can generative models collaborate with human musicians?
Yes, many generative models can work alongside human musicians to create collaborative pieces. AI systems can provide inspiration or assist in the composition process, leading to innovative musical outcomes.
8. What tools can I use to experiment with generative music?
Some popular tools include:
- OpenAI’s MuseNet
- Google’s Magenta
- AIVA (Artificial Intelligence Virtual Artist)
- Various software and plugins for sound design like Serum and Pure Data.
9. Are there ethical considerations when using generative models in music?
Yes, ethical considerations include issues of authorship, copyright, and the potential for AI to overlook cultural nuances in music, which can impact traditional musical forms and styles.
10. What is the future of generative models in music?
The future may see more advanced generative models capable of producing high-quality music, personalized musical experiences, enhanced interactive installations, and greater integration in music education.
Tips for Exploring Generative Models in Music
- Start with Open-Source Tools: Begin experimenting with free platforms like Google’s Magenta or OpenAI’s MuseNet to understand the capabilities of generative models without financial investment.
- Dive into Music Theory: A solid understanding of music theory will enhance your ability to evaluate and guide generative processes, leading to more coherent and engaging compositions.
- Experiment with Style Transfer: Use generative models to apply different styles to your compositions, allowing for creative cross-genre experimentation and novel soundscapes.
- Collaborate with AI: Consider co-creating music with AI tools, using them as partners in your creative process. This can lead to unexpected and innovative results.
- Engage in Community: Join online forums and communities focused on generative music to share insights, seek advice, and learn from others’ experiences.
- Focus on Quality Data: When training generative models, ensure you use high-quality and diverse datasets to improve the output quality and relevance of the generated music.
- Monitor Ethical Implications: Stay aware of the ethical considerations associated with AI-generated music, particularly regarding authorship and cultural sensitivity.
- Keep an Eye on Innovations: The field of generative music is rapidly evolving. Follow the latest research and developments to stay updated on new tools, techniques, and trends.
- Use Visualizations: Incorporate visualization tools to monitor the generative process, allowing for better understanding and fine-tuning of the models.
- Be Open to Experimentation: Embrace the experimental nature of generative music. Don’t hesitate to explore unconventional approaches and styles, as this can lead to new musical discoveries.