1. Introduction
Statistical methods form the backbone of data analysis and decision-making across various fields. Among these methods, the two predominant approaches are Bayesian and Frequentist statistics. Understanding the differences between these approaches is essential for researchers, data scientists, and statisticians, as the choice of method can significantly influence analysis outcomes. This article will compare and contrast the Bayesian and Frequentist approaches, elucidating their principles, applications, and practical implications.
2. Fundamental Concepts
2.1. What is the Frequentist Approach?
The Frequentist approach to statistics is based on the idea of long-run frequencies. In this framework, probability is interpreted as the limit of the relative frequency of an event occurring over many trials. Frequentist statistics focus on drawing conclusions from sample data without incorporating prior beliefs. Key characteristics include point estimation (single best estimate of a parameter) and the use of hypothesis testing to make inferences about populations.
2.2. What is the Bayesian Approach?
In contrast, the Bayesian approach incorporates prior beliefs or knowledge into the statistical analysis. Probability, in this context, is seen as a degree of belief or certainty about an event. Bayesian statistics allows for updating these beliefs based on new evidence, using Bayes’ theorem to compute posterior probabilities. This approach is characterized by the use of prior distributions, which represent initial beliefs about parameters, and posterior distributions, which are updated beliefs after observing data.
3. Key Differences Between Bayesian and Frequentist Approaches
3.1. Interpretation of Probability
- Frequentist: In this approach, probability is defined as the long-run frequency of events. For example, the probability of flipping a coin and getting heads is considered the ratio of heads to total flips as the number of flips approaches infinity.
- Bayesian: Probability is interpreted as a subjective degree of belief. For instance, one might say there is a 70% probability that it will rain tomorrow based on personal belief and prior knowledge.
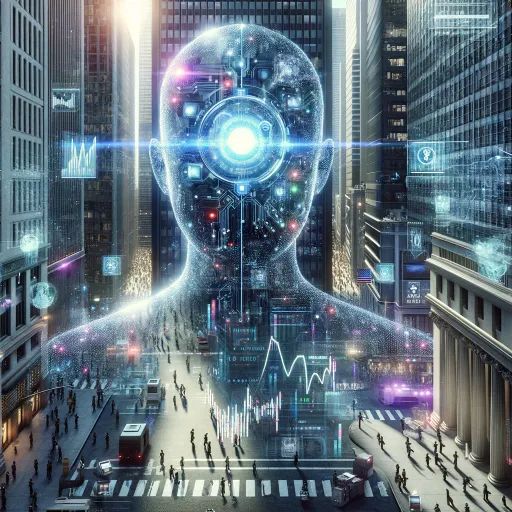
3.2. Role of Prior Information
- Frequentist: Prior information is not incorporated in analysis. Each study is treated independently, and conclusions are drawn solely from the data collected in the current experiment.
- Bayesian: Prior distributions are used to incorporate existing knowledge into the analysis. This allows researchers to combine prior beliefs with new data to make more informed inferences.
3.3. Parameter Estimation
- Frequentist: Parameters are estimated using point estimates, such as the sample mean. Confidence intervals provide a range of plausible values for the parameter, but they do not provide a direct probability for the parameter itself.
- Bayesian: Parameters are represented by distributions, specifically posterior distributions, which provide a complete picture of uncertainty. Credible intervals derived from these distributions give a range where the parameter is likely to fall, given the observed data.
3.4. Hypothesis Testing
- Frequentist: Hypothesis testing often relies on null hypothesis significance testing (NHST), where researchers determine whether to reject a null hypothesis based on p-values.
- Bayesian: Hypothesis testing is conducted using Bayes factors, which compare the likelihood of the observed data under different hypotheses, providing a probabilistic framework for making decisions.
4. Advantages and Disadvantages
4.1. Advantages of the Frequentist Approach
- Simplicity and Objectivity: The Frequentist approach is often viewed as more straightforward and objective, relying purely on the data at hand without prior assumptions.
- Established Methods: Frequentist methods are well-studied and widely accepted in various fields, making them a reliable choice for many analyses.
4.2. Disadvantages of the Frequentist Approach
- Limitations in Handling Prior Information: The Frequentist approach does not leverage prior knowledge, which can be a disadvantage in scenarios where such information is crucial.
- Misleading Results from p-values: P-values can be misinterpreted, leading to conclusions that do not accurately reflect the data’s significance.
4.3. Advantages of the Bayesian Approach
- Flexibility in Modeling: The Bayesian approach allows for the incorporation of prior information, enabling more nuanced models that reflect the complexities of real-world data.
- Incorporation of Prior Knowledge: By using prior distributions, Bayesian methods can produce more informed estimates and predictions.
4.4. Disadvantages of the Bayesian Approach
- Dependence on Prior Distributions: The choice of prior can significantly affect the results, and selecting an appropriate prior can be challenging.
- Computational Complexity: Bayesian methods can be computationally intensive, especially with large datasets, requiring advanced techniques such as Markov Chain Monte Carlo (MCMC) for inference.
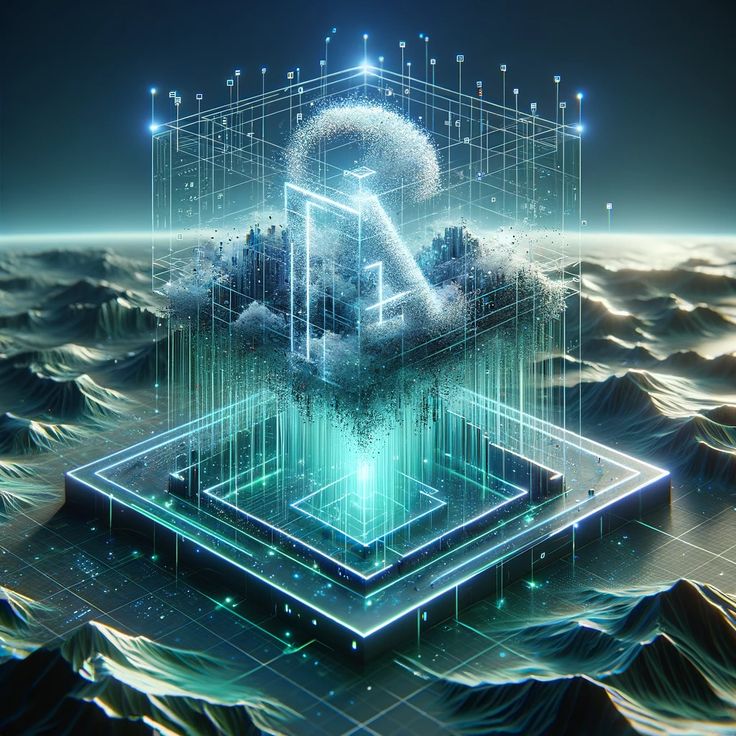
5. Applications in Various Fields
5.1. Medical Research
In clinical trials, the Frequentist approach is commonly used for hypothesis testing to determine the efficacy of new treatments. However, Bayesian methods are increasingly being applied to incorporate prior knowledge from previous studies, providing a more comprehensive analysis of treatment effects.
5.2. Machine Learning
Bayesian methods are prevalent in probabilistic modeling and machine learning, offering robust techniques for uncertainty estimation. Frequentist methods, such as traditional regression techniques, are often employed for hypothesis testing and model evaluation.
5.3. Social Sciences
In social sciences, both approaches are utilized for survey analysis and experimental design. Bayesian methods enable researchers to integrate prior knowledge about populations, while Frequentist methods provide a straightforward framework for testing hypotheses.
6. Practical Examples
6.1. Example of a Frequentist Analysis
Consider a clinical trial comparing a new drug to a placebo. A Frequentist analysis might involve calculating the mean difference in outcomes between groups and using a t-test to determine if the difference is statistically significant, reporting a p-value to support the conclusion.
6.2. Example of a Bayesian Analysis
In a similar trial, a Bayesian analysis would involve defining prior distributions for the treatment effect based on historical data. The observed data would then be used to update these priors, resulting in a posterior distribution that provides a range of probable treatment effects, along with credible intervals.
7. Conclusion
Understanding the differences between Bayesian and Frequentist approaches is crucial for selecting the appropriate statistical method based on the context of the analysis. While Frequentist methods offer simplicity and objectivity, Bayesian methods provide flexibility and the ability to incorporate prior knowledge. Researchers should consider the strengths and weaknesses of each approach to make informed decisions in their statistical analyses.
8. References
- A comprehensive list of academic papers, textbooks, and online resources for further reading on Bayesian and Frequentist approaches.
FAQs
1. What is the primary difference between Bayesian and Frequentist statistics?
The primary difference lies in the interpretation of probability. Bayesian statistics views probability as a degree of belief, incorporating prior information, while Frequentist statistics defines probability as the long-run frequency of events without considering prior knowledge.

2. When should I use the Bayesian approach?
You should consider using the Bayesian approach when you have prior knowledge or beliefs about a parameter that you want to incorporate into your analysis, or when you need to quantify uncertainty in a more flexible manner.
3. What are some common applications of Frequentist statistics?
Frequentist statistics is commonly used in various fields, including clinical trials, quality control, and survey analysis, where hypothesis testing and confidence intervals are essential.
4. What are credible intervals in Bayesian analysis?
Credible intervals provide a range of values within which a parameter is likely to fall, given the observed data. They differ from confidence intervals, which are defined in terms of long-run frequency properties.
5. What are some limitations of the Frequentist approach?
Frequentist statistics does not incorporate prior information, which can be a drawback in situations where such knowledge is available. Additionally, reliance on p-values can sometimes lead to misinterpretations of statistical significance.
6. What are Bayes factors?
Bayes factors are used in Bayesian hypothesis testing to compare the likelihood of the observed data under different hypotheses. They provide a probabilistic measure to evaluate which hypothesis is more plausible given the data.
7. How does Bayesian statistics handle uncertainty?
Bayesian statistics quantifies uncertainty using probability distributions, allowing for a comprehensive representation of beliefs about parameters. This contrasts with Frequentist statistics, which provides point estimates and confidence intervals.
8. Can I combine both approaches?
Yes, in practice, researchers may use both approaches to gain insights from different perspectives. Understanding the strengths and weaknesses of each can lead to more robust conclusions.
9. What tools or software can I use for Bayesian analysis?
There are several tools available for Bayesian analysis, including R (with packages like rstan
and brms
), Python (using libraries such as PyMC3 and TensorFlow Probability), and specialized software like Stan and JAGS.
10. Is one approach better than the other?
Neither approach is universally better; the choice depends on the specific context, data, and research questions. Understanding both frameworks allows researchers to choose the most suitable method for their analyses.
Tips for Understanding and Applying Bayesian and Frequentist Approaches
- Familiarize Yourself with Key Concepts: Understand the fundamental principles of both approaches, including probability interpretations, parameter estimation, and hypothesis testing.
- Practice with Real Data: Apply both Bayesian and Frequentist methods to real datasets to see how the results differ. This hands-on experience can help solidify your understanding.
- Study Examples: Analyze case studies or examples that illustrate the application of both approaches in various fields, such as healthcare, finance, and social sciences.
- Explore Software Tools: Experiment with statistical software that supports both Bayesian and Frequentist analyses. Familiarity with these tools will enhance your ability to conduct complex analyses.
- Engage with the Community: Join online forums, discussion groups, or courses focused on Bayesian and Frequentist statistics. Engaging with others can provide insights and resources that enrich your understanding.
- Stay Updated: The fields of statistics and data science are rapidly evolving. Keep abreast of the latest research, techniques, and software developments related to both Bayesian and Frequentist methods.
- Document Your Work: When performing analyses, document your methodology and decisions, especially regarding the choice of priors in Bayesian analysis. This practice aids in transparency and reproducibility.
- Consider the Context: Always consider the specific context of your analysis when choosing between Bayesian and Frequentist methods. The nature of your data and the research questions can significantly influence the choice of approach.
- Consult Experts: If you are unsure which method to use, consider consulting with a statistician or data scientist experienced in both Bayesian and Frequentist approaches. Their expertise can provide valuable guidance.
- Be Open to Learning: Both Bayesian and Frequentist approaches have their merits. Be open to learning from both perspectives and integrating them into your statistical toolbox.