The integration of deep learning into healthcare has revolutionized medical practices, from diagnostics to personalized treatments. By leveraging vast amounts of data, deep learning models are able to identify complex patterns and generate insights that would be difficult for human experts to detect alone. This article explores the wide-ranging applications of deep learning in healthcare and how these technologies are transforming the industry.
1. Medical Imaging
One of the most prominent applications of deep learning in healthcare is in medical imaging. Deep learning models, especially convolutional neural networks (CNNs), are used to analyze various types of medical scans such as X-rays, MRIs, and CT scans.
Image Classification and Detection
Deep learning algorithms are adept at classifying images and detecting abnormalities, such as tumors, fractures, and lesions. These models can assist radiologists in identifying early signs of cancer, heart disease, and other critical conditions.
Segmentation and Enhancement
Deep learning is also used for segmentation, where specific regions of interest (such as cancerous tissues or damaged areas) are automatically highlighted. These technologies enhance the precision of diagnostics, reducing human error and improving patient outcomes.
Examples
- Early cancer detection: Deep learning models can detect lung cancer nodules or breast cancer cells earlier and more accurately than traditional methods.
- Automated pathology analysis: AI tools are capable of analyzing histopathological images to detect diseases like prostate cancer.
2. Drug Discovery and Development
Deep learning has significantly accelerated the traditionally slow and expensive process of drug discovery and development.
Accelerating Drug Discovery
By predicting molecular interactions and analyzing the vast landscape of potential compounds, deep learning models can identify promising drug candidates faster than manual approaches.
Reducing Costs and Time
Deep learning models can simulate parts of clinical trials and predict drug efficacy, potentially reducing the need for costly and time-consuming phases in drug development.
Examples
- Drug repurposing: Deep learning has been used to explore new applications for existing drugs, such as identifying potential treatments for COVID-19.
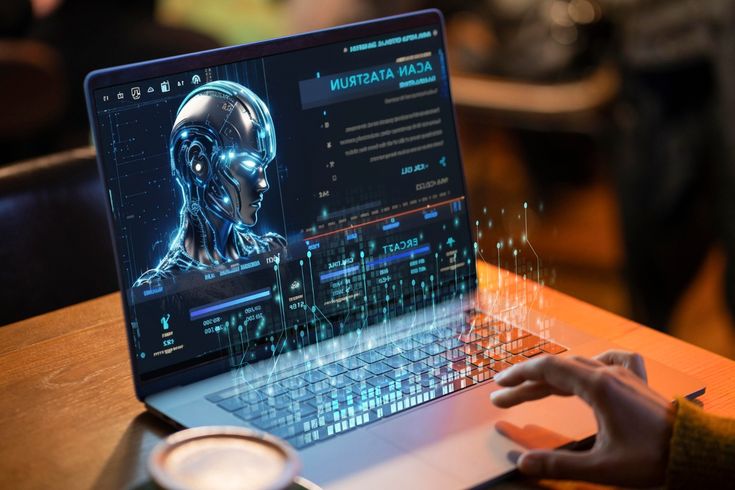
3. Personalized Medicine
Personalized medicine aims to tailor treatments to individual patients based on their unique genetic and health data. Deep learning plays a pivotal role in making this vision a reality.
Predictive Analytics for Treatment
By analyzing patient histories, genetic profiles, and other data, deep learning models can predict which treatments are most likely to succeed for specific individuals, enhancing the effectiveness of medical interventions.
Genomic Data Analysis
Deep learning has transformed genomic analysis by identifying gene mutations and predicting the risk of genetic diseases. This data helps physicians design personalized treatment plans and preventive measures.
Examples
- Cancer treatment: Deep learning can help develop customized chemotherapy regimens based on individual patient profiles, improving outcomes and reducing side effects.
4. Predictive Analytics and Early Disease Detection
Predictive analytics in healthcare uses historical data to anticipate future health outcomes, such as the likelihood of disease onset.
Risk Prediction
Deep learning models analyze patient data (e.g., lifestyle factors, lab results) to predict the risk of diseases such as diabetes, heart disease, or stroke. This allows healthcare providers to take preventive action before symptoms manifest.
Early Diagnosis
In addition to risk prediction, deep learning models can detect diseases in their early stages by analyzing subtle patterns in patient data, such as brain scans for early signs of Alzheimer’s disease.
Examples
- Early detection of Alzheimer’s: Deep learning models analyze brain scans and cognitive assessments to predict the onset of Alzheimer’s disease years before clinical symptoms appear.
5. Natural Language Processing (NLP) in Healthcare
Natural language processing (NLP) is a subset of deep learning that processes and understands human language. In healthcare, it is used to analyze unstructured text from medical records and research papers.
Processing Medical Records
Deep learning models can parse and interpret large amounts of unstructured data from Electronic Health Records (EHRs), enabling healthcare providers to gain insights and improve decision-making.
Clinical Decision Support
By analyzing vast amounts of medical literature, NLP models help physicians stay informed about the latest treatments and guidelines, making clinical decision support faster and more accurate.
Chatbots and Virtual Assistants
AI-driven chatbots and virtual assistants powered by NLP help patients with scheduling, symptom checking, and even mental health support, improving patient engagement and reducing administrative burdens.
6. Robotics and Surgery Assistance
Deep learning is powering the next generation of robotics, particularly in surgical applications.
AI-Assisted Surgery
Robotic surgical systems, such as the da Vinci Surgical System, use deep learning algorithms to enhance the precision of surgeries. These systems provide real-time assistance to surgeons by analyzing data and improving the accuracy of procedures.
Real-Time Data Analysis During Surgery
Deep learning models monitor patient data during surgery, such as vital signs and organ images, to help surgeons make real-time decisions.
Examples
- Robotic surgery systems: These systems offer enhanced precision for complex procedures like heart surgery and neurosurgery, reducing recovery times and improving patient outcomes.
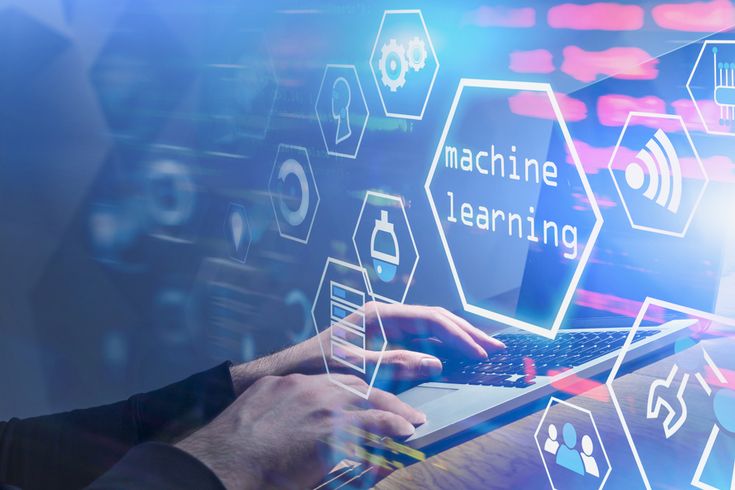
7. Wearable Devices and Remote Monitoring
Wearable devices like smartwatches and fitness trackers have opened up new possibilities for continuous patient monitoring.
Analyzing Data from Wearables
Deep learning algorithms can interpret data from wearable devices, such as heart rate or sleep patterns, to detect anomalies and predict potential health issues.
Continuous Patient Monitoring
Wearables combined with deep learning enable remote monitoring of patients with chronic conditions, such as heart disease or diabetes, allowing for timely interventions based on real-time data.
Examples
- Early detection of arrhythmias: Smartwatches equipped with AI algorithms can detect irregular heartbeats and alert users to seek medical attention before severe symptoms arise.
8. Mental Health and Behavioral Analysis
Deep learning is also making strides in mental health by analyzing behavioral data, such as speech patterns, facial expressions, and online activity.
Emotion and Mood Detection
Deep learning models can detect emotional states and identify signs of mental health disorders like depression, anxiety, or PTSD by analyzing patterns in speech and facial cues.
Treatment Recommendations
AI models can recommend treatment plans for mental health conditions based on patient history, clinical data, and current emotional states.
Examples
- AI-based mental health platforms: These platforms use deep learning to monitor users’ moods and provide early intervention for mental health crises.
9. Challenges and Ethical Considerations
While deep learning offers many benefits in healthcare, it also raises important challenges and ethical considerations.
Data Privacy and Security
The use of deep learning in healthcare involves processing sensitive patient data, which raises concerns about privacy and the security of medical records.
Bias and Fairness in Algorithms
Deep learning models can sometimes inherit biases from the data they are trained on, leading to unequal treatment or incorrect diagnoses for certain patient populations. Ensuring fairness and removing bias in AI systems is crucial for maintaining trust in healthcare applications.
Regulatory Hurdles
Before deep learning applications can be widely adopted in healthcare, they must meet strict regulatory standards to ensure patient safety. For example, AI models for medical diagnostics must undergo thorough testing and approval by regulatory bodies like the FDA.
Explainability of Deep Learning Models
Deep learning models, particularly deep neural networks, are often considered “black boxes” because it is difficult to interpret how they make decisions. In healthcare, where explainability is crucial, this lack of transparency can be problematic.
10. Future of Deep Learning in Healthcare
The potential for deep learning in healthcare is vast. As AI models become more sophisticated and data availability increases, deep learning will likely play a central role in precision medicine, enabling personalized treatments and improving patient care across the board.
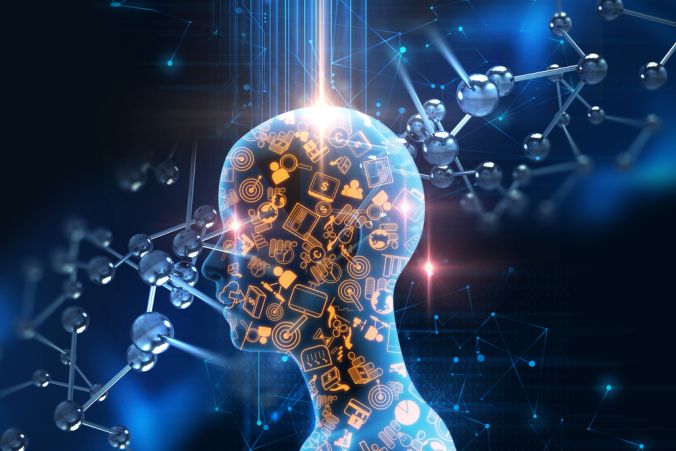
Potential for Advancing Precision Medicine
Deep learning has the potential to refine precision medicine further, leading to more targeted treatments for complex diseases.
Integration of AI with Healthcare Professionals
Rather than replacing human doctors, AI will increasingly assist healthcare professionals by offering advanced decision support, enabling them to make more accurate diagnoses and treatment decisions.
11. Conclusion
The applications of deep learning in healthcare are already making a significant impact on how diseases are diagnosed, treated, and monitored. From medical imaging and drug discovery to personalized medicine and mental health analysis, deep learning is transforming the healthcare landscape. While challenges remain, such as data privacy and regulatory approval, the future of AI in healthcare is incredibly promising, with the potential to improve outcomes, reduce costs, and save lives.
FAQs: Applications of Deep Learning in Healthcare
1. What is deep learning and how is it used in healthcare?
Deep learning is a subset of artificial intelligence (AI) that uses neural networks to model and interpret complex patterns in data. In healthcare, it’s used for tasks like medical imaging, drug discovery, personalized medicine, and predictive analytics.
2. How does deep learning improve medical imaging?
Deep learning algorithms analyze medical images like X-rays, CT scans, and MRIs to detect abnormalities, such as tumors or fractures, with greater accuracy and speed than traditional methods.
3. How is deep learning applied in drug discovery?
Deep learning accelerates drug discovery by predicting molecular interactions, identifying drug candidates, and simulating clinical trials. This can reduce the time and cost associated with developing new drugs.
4. What role does deep learning play in personalized medicine?
Deep learning helps tailor treatments to individual patients by analyzing genetic data and health records to predict treatment outcomes, optimizing drug doses, and designing customized treatment plans.
5. How can deep learning assist in early disease detection?
Deep learning models analyze patient data to predict the risk of diseases such as cancer, Alzheimer’s, and cardiovascular conditions, often detecting them in early stages before symptoms appear.
6. What are the uses of deep learning in wearable devices and remote monitoring?
Deep learning algorithms analyze data from wearables (e.g., heart rate, blood oxygen levels) to monitor health in real-time, providing early warnings for issues like arrhythmias or sleep apnea.
7. Can deep learning help with mental health diagnosis?
Yes, deep learning models analyze speech, facial expressions, and behavior to detect mood disorders and other mental health conditions, enabling early diagnosis and recommending treatment options.
8. What are the challenges of using deep learning in healthcare?
Challenges include ensuring data privacy and security, addressing bias in algorithms, navigating regulatory hurdles, and making AI models explainable so healthcare professionals understand the rationale behind decisions.
9. How is deep learning used in robotic surgery?
AI-powered robotic systems assist surgeons with precision and real-time data analysis, making complex surgeries safer and more accurate.
10. What is the future potential of deep learning in healthcare?
The future of deep learning in healthcare includes advancements in precision medicine, improved diagnostic tools, and deeper integration of AI with human healthcare professionals, leading to better patient outcomes.
Tips: Leveraging Deep Learning in Healthcare
1. Start with High-Quality Data
- Ensure your healthcare datasets are clean, well-structured, and free from bias. Deep learning models require high-quality data to generate accurate and reliable results.
2. Focus on Data Privacy and Security
- Protect patient data by using secure platforms, encrypting sensitive information, and complying with regulatory frameworks like HIPAA to safeguard privacy.
3. Use Pre-trained Models Where Possible
- Take advantage of existing pre-trained models for healthcare applications, such as medical image classification, to save time and resources during development.
4. Combine Deep Learning with Human Expertise
- AI in healthcare should complement human expertise, not replace it. Use AI tools to enhance decision-making while leaving critical decisions to healthcare professionals.
5. Understand the Difference Between Additive and Multiplicative Models
- In predictive analytics, choose the right model for your data. Additive models work well when the seasonal effect is constant, while multiplicative models are better when seasonal variations grow with time.
6. Ensure Algorithm Transparency
- Choose or design deep learning models that provide explainable outputs, especially in critical healthcare scenarios. Doctors need to trust AI decisions and understand how they are made.
7. Monitor Model Performance
- Regularly update and retrain your models with new data to maintain accuracy and adapt to changing healthcare environments or patient demographics.
8. Apply Deep Learning for Early Detection
- Use deep learning for early disease detection by analyzing subtle patterns in medical data that are hard for humans to detect, allowing for earlier interventions and better outcomes.
9. Implement Continuous Learning Systems
- In applications like wearable health monitoring, ensure your deep learning system can continuously learn and adapt to new data in real-time, improving its predictive abilities.
10. Consider Ethical Implications
- Address potential biases in your models, ensure fairness in healthcare delivery, and always consider the ethical implications of AI-driven decisions in healthcare settings.