Generative models have revolutionized the field of artificial intelligence, enabling machines to create realistic data in various domains, including images, audio, and text. As research in this area continues to evolve, it’s essential to explore the future trends that will shape the development and application of generative models. This article discusses anticipated advancements in model architectures, training techniques, cross-domain applications, ethical considerations, and the role of community collaboration in generative model research.
1. Introduction
Generative models have gained prominence in recent years, transforming the landscape of machine learning. From generating realistic images to creating human-like text, these models have showcased their potential across various applications. Understanding the trajectory of generative model research is crucial for researchers and practitioners looking to leverage these advancements effectively. This article aims to highlight future trends that will influence the direction of generative model research.
2. Advances in Model Architectures
A. Development of Hybrid Models
The future of generative modeling lies in the development of hybrid models that combine the strengths of various architectures, such as GANs (Generative Adversarial Networks) and VAEs (Variational Autoencoders). By integrating these approaches, researchers can enhance the quality and diversity of generated outputs. Hybrid architectures can lead to improved performance by leveraging the unique advantages of each model type.
B. Self-Supervised Learning
Self-supervised learning, which utilizes unlabeled data for training, is poised to play a significant role in generative model research. By allowing models to learn from vast amounts of unlabeled data, self-supervised approaches can lead to better generalization and improved performance. This trend can make generative models more accessible and applicable across various domains.
C. Attention Mechanisms and Transformers
The rise of transformer-based architectures has transformed generative modeling. These models leverage attention mechanisms to capture long-range dependencies in data, enabling them to produce high-quality outputs. The application of transformers in generative models is expected to expand, leading to even more sophisticated and versatile applications.
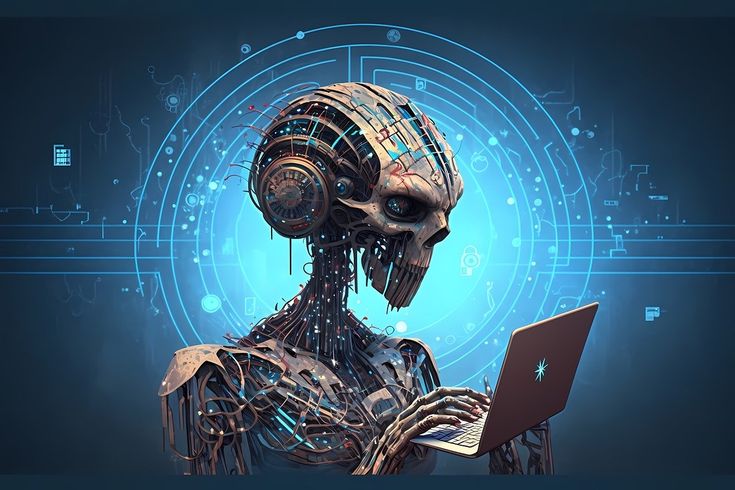
3. Improved Training Techniques
A. Enhanced Optimization Strategies
Future generative models will benefit from enhanced optimization strategies aimed at faster convergence and improved stability. Techniques to address issues like mode collapse in GANs will become increasingly important, enabling models to produce diverse outputs without sacrificing quality.
B. Curriculum Learning
Curriculum learning, which involves gradually increasing the complexity of tasks during training, is gaining traction in generative model research. By starting with simpler tasks and progressing to more complex ones, models can become more robust and better equipped to handle real-world challenges.
C. Federated Learning
Federated learning, which allows for collaborative model training across decentralized data sources, will emerge as a key trend in generative model research. This approach enhances privacy and data security, as models can learn from diverse datasets without exposing sensitive information. The implications of federated learning are significant, especially in fields like healthcare and finance.
4. Cross-Domain Applications
A. Generative Models in Healthcare
Generative models are set to play a transformative role in healthcare. Applications in medical imaging, drug discovery, and personalized medicine are on the rise. However, ethical considerations, such as data privacy and the potential for biased outputs, must be addressed to ensure responsible use in this critical domain.
B. Use in Creative Industries
The creative industries are increasingly embracing generative models for applications in music, art, and content generation. These models facilitate human-AI collaboration, enabling artists and creators to explore new creative avenues while retaining control over their work.
C. Role in Scientific Research
Generative models are becoming indispensable in scientific research, providing tools for data generation in simulations and experiments. By generating synthetic data, researchers can enhance scientific discovery, accelerate experimentation, and explore hypotheses that would otherwise be difficult to test.
5. Addressing Ethical and Societal Implications
A. Bias and Fairness in Generative Models
As generative models become more widespread, addressing bias and fairness will be critical. Researchers must identify and mitigate biases present in training data to ensure that generated outputs are equitable and representative of diverse populations.
B. Transparency and Interpretability
The need for transparency and interpretability in generative models is gaining attention. Researchers are developing tools and techniques to make generative models more explainable, enabling stakeholders to understand how and why specific outputs are generated. This transparency is vital for fostering trust in AI systems.
C. Regulation and Governance
As generative models continue to advance, frameworks for regulation and governance will be essential. Establishing guidelines for responsible AI use can help mitigate potential risks associated with generative models, including misuse in areas like deepfakes and misinformation.

6. The Role of Community and Collaboration
A. Open-Source Initiatives
Open-source initiatives are vital for the progress of generative model research. Community contributions enable researchers to share models, datasets, and findings, accelerating innovation and collaboration across the field.
B. Interdisciplinary Collaboration
Future advancements in generative models will benefit from interdisciplinary collaboration between AI researchers, ethicists, domain experts, and creative practitioners. This cross-pollination of ideas and techniques will lead to more robust and responsible generative models.
7. Conclusion
The future of generative model research is filled with exciting possibilities, from advancements in model architectures and training techniques to cross-domain applications and ethical considerations. As researchers and practitioners navigate this evolving landscape, staying informed and adaptable will be essential for harnessing the full potential of generative models. By embracing these trends, the community can ensure that generative models contribute positively to society and drive innovation across various fields.
8. References
- Key literature on generative models
- Research papers and articles for further reading
- Online resources and communities for practitioners exploring generative models
FAQs About Future Trends in Generative Model Research
1. What are generative models?
Generative models are a class of machine learning models that can create new data instances that resemble training data. Examples include Generative Adversarial Networks (GANs) and Variational Autoencoders (VAEs).
2. Why is research into generative models important?
Research in generative models is crucial because these models can generate realistic data across various domains, enabling advancements in areas such as healthcare, art, and scientific research. They also facilitate creative collaborations between humans and machines.
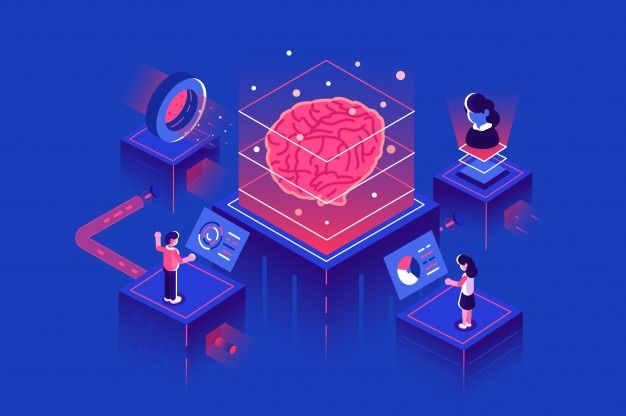
3. What are some anticipated advancements in model architectures?
Future advancements may include hybrid models that combine the strengths of different architectures, self-supervised learning techniques, and the integration of attention mechanisms and transformers, which enhance the model’s ability to generate high-quality outputs.
4. How will training techniques improve in generative models?
Improvements in training techniques may involve enhanced optimization strategies to reduce mode collapse, the implementation of curriculum learning for better model robustness, and the adoption of federated learning for privacy-preserving model training.
5. In which domains are generative models expected to have the most impact?
Generative models are expected to significantly impact healthcare, creative industries (such as art and music), and scientific research, providing tools for data generation, enhancing creativity, and accelerating scientific discovery.
6. What ethical considerations are associated with generative models?
Key ethical considerations include addressing bias and fairness in generated outputs, ensuring transparency and interpretability of models, and establishing regulations to govern responsible AI use, especially in sensitive areas like deepfakes and misinformation.
7. How can researchers and practitioners collaborate effectively in this field?
Collaboration can be fostered through open-source initiatives that allow sharing of models, datasets, and findings. Interdisciplinary collaboration among AI researchers, ethicists, and domain experts can lead to more robust and responsible applications of generative models.
8. What resources are available for learning more about generative models?
Numerous resources are available, including research papers, online courses, tutorials, and active online communities focused on AI and machine learning. Staying engaged with these resources is essential for keeping up with advancements.
Tips for Engaging with Future Trends in Generative Model Research
- Stay Informed: Regularly read research papers and articles to keep up with the latest developments and breakthroughs in generative models.
- Participate in Online Communities: Engage with online forums, social media groups, and platforms like GitHub to share knowledge, ask questions, and collaborate on projects.
- Experiment with Open Source Tools: Explore open-source libraries and tools for generative modeling, such as TensorFlow, PyTorch, and Keras. Practical experience will deepen your understanding.
- Attend Conferences and Workshops: Participate in AI and machine learning conferences to network with experts, share insights, and learn about cutting-edge research in generative models.
- Focus on Ethical AI Practices: Be proactive in addressing ethical considerations by incorporating bias mitigation techniques and promoting transparency in your generative models.
- Collaborate Interdisciplinarily: Seek opportunities to work with experts from other fields, such as art, healthcare, or ethics, to broaden your perspective and enhance your models’ applicability.
- Document Your Findings: Keep detailed records of your experiments, methodologies, and results. Documentation is vital for reproducibility and sharing knowledge with others.
- Embrace Continuous Learning: The field of generative modeling is dynamic. Stay open to new ideas, techniques, and approaches to adapt to the evolving landscape.
- Test Diverse Applications: Explore different applications of generative models beyond traditional domains. This exploration can lead to innovative uses and insights.
- Be Patient and Iterative: Generative model research often involves trial and error. Be patient, learn from failures, and iteratively refine your models based on evaluation and feedback.